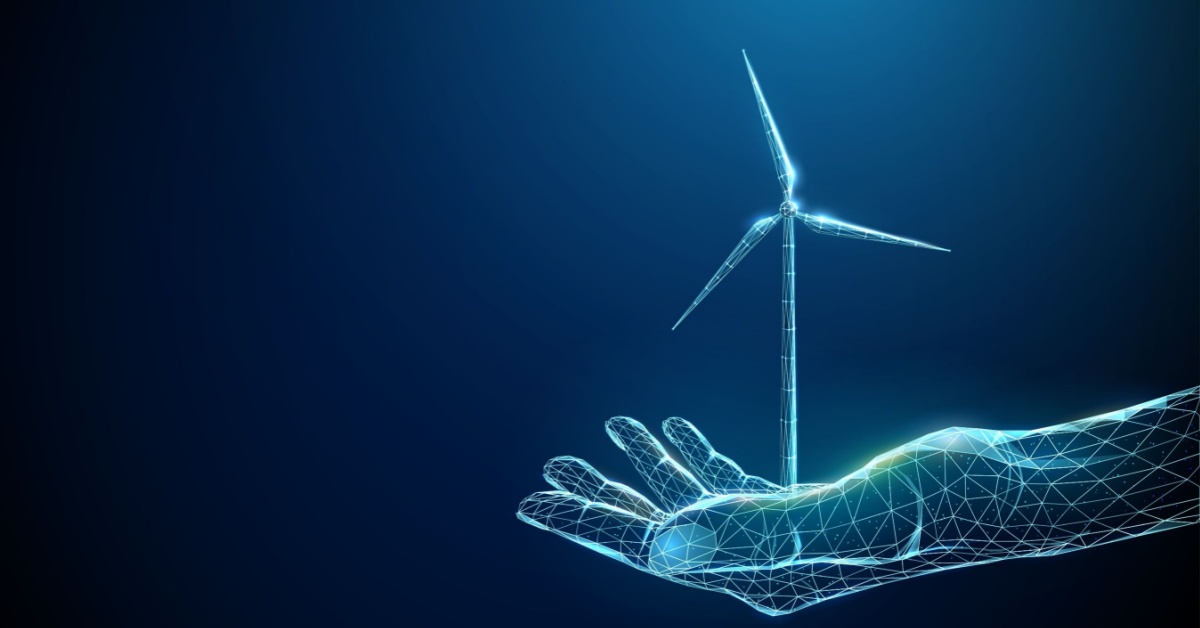
In the competitive investment landscape of clean tech, startups are vying for attention. For years, AI has been the ultimate buzzword for those seeking to raise funding. Investors have been inundated with pitches promising “AI-driven” solutions, making it increasingly difficult to separate true innovation from optimistic branding.
Artificial intelligence is a powerful enabler in clean tech sectors such as virtual power plants (VPPs), distributed energy resource management systems (DERMS), electric mobility and vehicle-to-grid (V2G) solutions. When applied correctly, AI can optimize resources, drive efficiency and make systems smarter for the mobility, utility and building/home energy management industries. However, not every company claiming AI is building it in a meaningful way.
In the previous decade, many clean tech startups felt compelled to claim that their solutions were AI-driven even when they were not. Exaggerating their AI capabilities, many companies invested in just enough AI to pass the “straight face” test. This created the illusion of technical innovation when companies were actually relying on basic algorithms or glorified spreadsheets that were neither novel nor scalable. This illusion was not necessarily nefarious but rather a gross underestimation of what it takes to develop and scale a truly robust AI platform.
However, the landscape has shifted dramatically in the past year. The rapid advancement of AI, particularly with the emergence of large-scale foundational models from OpenAI and others, has changed the game. Today, many startups no longer need to develop AI from the ground up. Instead, they can integrate powerful, pre-trained models with minimal effort, making AI more accessible. This democratization of AI has both leveled the playing field and made it harder than ever for investors to assess which companies are truly differentiated.
In 2025, the key question for investors is no longer whether a startup is using actual AI, as it almost certainly is, but rather how it is using AI
In 2025, the key question for investors is no longer whether a startup is using actual AI, as it almost certainly is, but rather how it is using AI and whether it can create lasting value and differentiation. This distinction falls into two categories:
- Startups tackling complex challenges that require deep, in-house AI development. These ‘Deep AI Innovators’ must build proprietary AI capabilities to achieve their goals.
- Startups exclusively leveraging existing AI models with modest additional development, what can be called the ‘competitive wrapper’ phenomenon. Here differentiation will come from proprietary data, distribution channels or other strategic advantages.
Deep AI innovators
Investors can take several steps to identify true deep AI innovators in tech-heavy applications such as VPPs, which pose sophisticated and specific technical challenges. VPPs are complex applications requiring significant investment in AI to predict energy supply and varied demand profiles, integrate renewable energy sources, optimize grid stability\and manage energy systems more efficiently.
The team’s expertise is one of the clearest indicators in evaluating deep AI innovators. A company that has invested heavily in AI should have data scientists and machine learning engineers at its core, not just on the periphery. But having the team in place is not enough. Investors should scrutinize whether the data science team’s efforts are actively being embedded in the software platform and not just serving as a ‘research’ arm. The danger is that the software platform and developed AI capabilities do not mesh or are unable to be fully integrated. Evaluation should focus on whether the integrated AI platform is robust enough to move from small-scale demonstrations to larger deployments. Companies that rely on manual intervention behind the scenes (what’s known as “Wizard of Oz” operations) will struggle to scale.
The consequences of investing in deep AI innovation start-ups with exaggerated AI claims can be significant. Investors may find themselves stuck with a product that cannot scale, resulting in wasted capital and lost market opportunities. Conversely, companies with real AI innovations often offer higher returns, as they can deliver on the promise of smarter, more efficient systems. A solid, scalable AI-driven platform is versatile. Such platforms in nascent markets can be repurposed for nearer-term revenue. For example, an AI-driven V2G platform can find revenue in a VPP application or DR market application while seeding the ground for V2G to enable EVs to hit the market
Competitive wrapper startups
The explosion of AI-powered applications will continue across industries where companies leverage existing large language models (LLMs) with modest additional development. For instance, real estate platforms can use AI to automate property descriptions, and electricians can leverage AI-powered diagnostics. In clean tech, predictive maintenance for renewable assets could be one such application. AI-driven energy audits could help homeowners and businesses identify inefficiencies, while automated carbon accounting tools could streamline sustainability reporting. These are all viable products, but how do companies prevent the next version of the LLM from obsoleting their offer? This is a fundamental distinction that investors should understand when evaluating these competitive wrapper startups.
With AI becoming table stakes, startups must develop true competitive advantages beyond merely integrating existing models. They need to shift their evaluation framework from asking, “Is this company really using AI?” to “Is this company using AI in a way that creates sustainable value that is scalable and necessary?”
Some AI applications sound impressive but don’t provide a meaningful advantage over traditional methods. AI capabilities should be integral to the business model, not just a feature. Most importantly, investors need to be convinced that the startup in question has not only mitigated risk from progressively more intelligent AI-based models but is structured in a way that builds on them as they will evolve at a rapid rate. Its long-term prospects are weak if a company’s core differentiation is based on capabilities that will soon be offered natively by foundational model providers.
Investors can help insulate their investment choices from this risk by looking for differentiation in key areas such as access to unique data sets, channel and distribution strategy, and proprietary hardware integration.
Questions investors should ask
AI models are only as good as the data they are trained on. Investors should ask if the company owns or generates proprietary data. If the AI models depend entirely on publicly available or third-party data, the competitive advantage may be weak. Startups with proprietary datasets can build AI models that outperform generic solutions. This is especially true in clean tech, where historical grid performance, weather data and building energy use are crucial for optimizing economics and efficiencies.
Investors also should determine if the company has exclusive partnerships, a strong brand, or regulatory advantages. Given that AI-based solutions are increasingly commoditized, companies with strong channel access, exclusive partnerships and regulatory inroads have a strategic edge. This is particularly true in the utility and mobility sectors, which tend to be more conservative when it comes to software-related infrastructure and perceived technology risk. For example, a start-up with pre-existing utility partnerships for a demand response play will have a much stronger advantage than one simply offering an AI-powered energy management tool.
Subscribe to the free Energy Changemakers Newsletter.
While software-based AI solutions are easily replicable, those embedded in proprietary hardware provide more of a moat, even if only in the near term. They offer edge computing and build a more defensible market position. Proprietary hardware also generates a path to early revenue while the product scales to generate meaningful SaaS revenue. It can provide differentiation that pure software solutions cannot.
By honing their evaluation criteria for deep AI innovators and competitive wrapper companies, investors can better identify the clean tech startups poised to deliver real, defensible innovation in an AI-saturated world. By sorting fact from fiction early in the investment process, investors mitigate risk and position themselves to back companies that will drive the future of clean tech.
Anna Demeo, managing partner at Climate Tech Strategy Advisors, acts as a strategic and technical advisor for companies and investors across the cleantech sector.