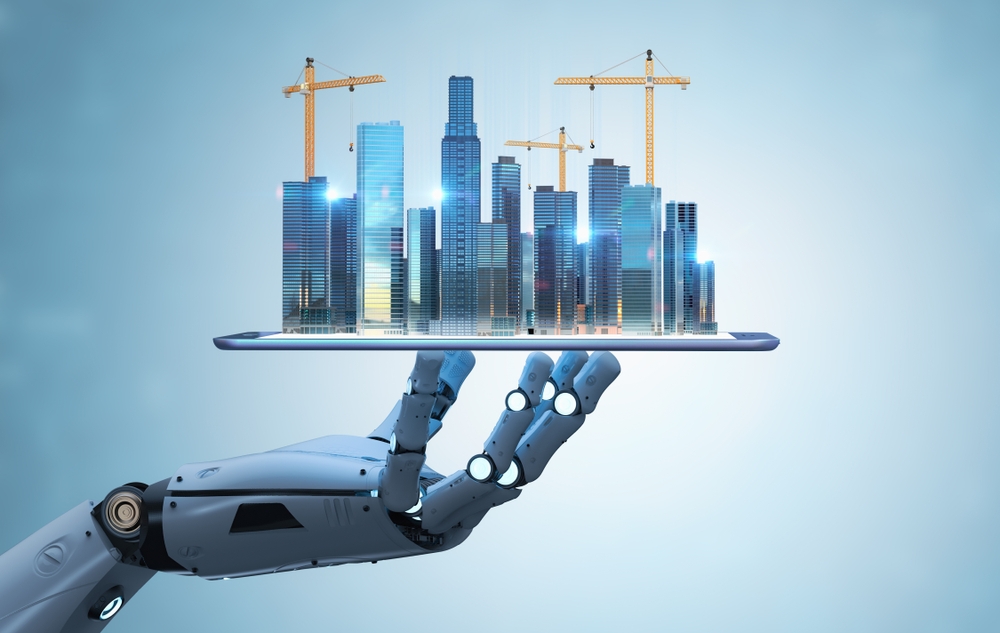
Guest contributor Anna Demeo describes how to recognize true AI-driven software and its use in virtual power plants.
Virtual power plants (VPPs) are increasingly critical to tackle climate change and strengthen energy resilience. However, deploying true AI-driven software is the key to unlocking their full potential.
VPPs do not rely on a single energy source. Instead, they harness a diverse network of distributed energy resources (DERs), including solar panels, batteries, smart devices, and electric vehicles (EVs). Each distributed resource possesses unique characteristics and constraints, necessitating sophisticated AI integration for optimal management and to leverage their collective output.
As the variety and number of DERs grow, so does the complexity of their management.
Robust AI-driven platforms can process data from diverse sources, understand and predict patterns of energy usage and power generation, and then make real-time decisions to optimize resources for a virtual power plant. This level of sophistication in AI is essential for both the efficient operation of individual DERs and the overall reliability and stability of the power grid.
To achieve this, the industry must extend well beyond simple rule-based algorithms that have been the hallmark of early software platforms in this space. Rule-based algorithms use predefined rules or logic to make decisions. These rules limit them, and they cannot learn from new data or adapt to changing environments, which are critical in energy and transportation applications.
For example, a software platform for a residential battery may employ a rule-based algorithm to discharge to the grid when utility rates are high to save money for the homeowner. But if that same battery also participates in a demand response program and must discharge on a utility-triggered event, which rule should be followed? The answer varies based on the time of day, season, program requirements, and other constraints a simple rule-based model cannot navigate.
Deterministic models offer more sophistication, using machine learning and decision trees to predict a specific outcome based on input data. While more suitable for the battery example, they, too, fall short when systems get more complicated as they assume no randomness in the future events they predict. On the other hand, probabilistic forecasting acknowledges uncertainty and randomness in future events. It provides a range of possible outcomes along with probabilities for each outcome. Such a model can learn from data, adapt, and improve over time, which is the real power of AI. Integrating this level of AI is crucial in leveraging the flexibility and variability inherent to a virtual power plant.
The predictive capabilities of AI are valuable in managing uncertainty and, therefore, particularly useful in scenarios like energy markets where numerous variables can affect future events. By analyzing vast datasets, AI-driven software can identify patterns and predict potential disruptions based on global trends, weather patterns, and historical data. Electricity prices, for example, can vary based on demand, supply, weather, and other market factors. An AI-driven VPP can create predictive models based on probabilities of potential future outcomes. This will allow it to effectively respond to price signals in real-time, making decisions about when to store, consume, or sell back energy to the grid. This capability is crucial for maximizing economic benefits for providers and DER owners and maintaining the cost-effectiveness of the VPP.
Electrification of transportation is the ultimate use case for AI in VPPs. EVs are poised to constitute a growing segment of distributed energy resources, adding yet another layer of complexity. When equipped with vehicle-to-grid (V2G) technology, EVs draw power from the grid and supply power back. This bidirectional capability turns EVs into mobile energy storage units.
The sheer volume of EVs estimated over the next decade provides the potential of gigawatts of storage for a grid that desperately needs it. However, unlike stationary storage, the availability of mobile storage as a grid resource is not constant, as a vehicle’s primary use is transportation. Even when available and plugged in, vehicle owners will want to protect some minimum amount of driving range, translated as state of charge of the batteries.
These factors, along with the health of the battery and vehicle warranty considerations, add to the complexity of managing EV resources within a VPP. This myriad of constraints and uncertainty require scalable models that employ probabilistic forecasting and machine learning so that the VPP can continuously predict, adapt and learn from variables such as telemetry data, electricity rate structures, driving patterns, user preferences and weather patterns.
AI is the term of the day and is being applied increasingly in marketing campaigns. So, as more companies claim to integrate AI into their solutions, it’s important to discern their actual level of sophistication and scalability. True AI offers significant advantages in handling complexity, adapting to new data, and providing valuable insights in uncertain scenarios. The gap between basic automation and genuine, scalable AI will become increasingly evident as the technology advances, underscoring the need for substantial investment in real AI capabilities for businesses seeking to stay competitive in the digital age.
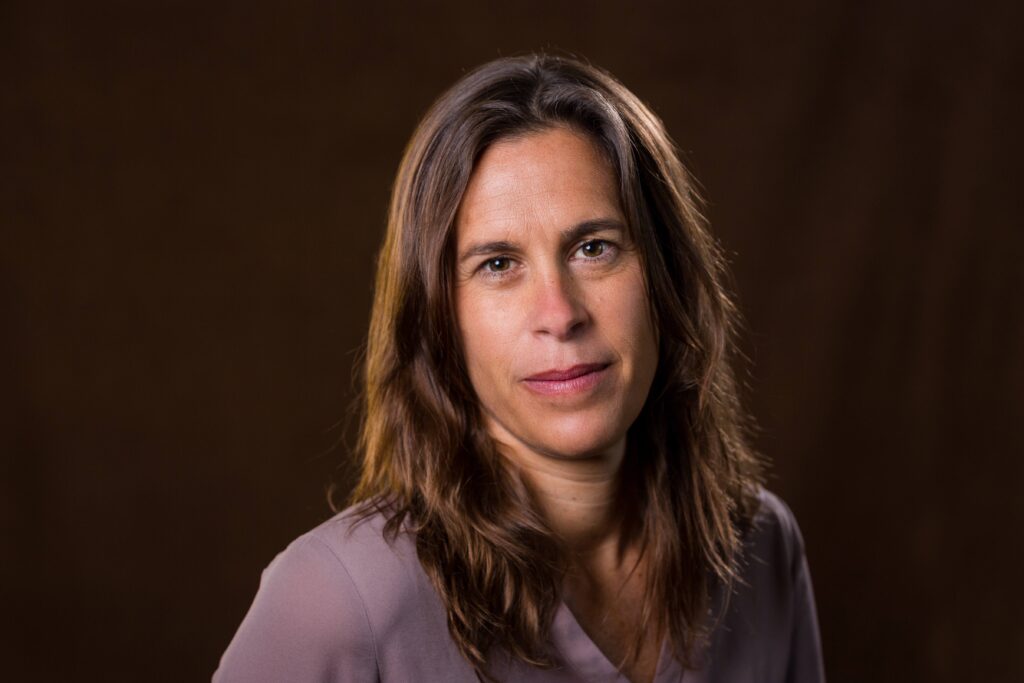
Anna Demeo is a strategic and technical advisor for companies and investors across the cleantech sector.